What is Data Science Process?
Data science has emerged as a crucial field in the digital era, enabling organizations to make data-driven decisions and gain valuable insights. The Steps in data science process involve a series of well-defined steps that help extract meaningful information from raw data. Steps in data science process are exactly like extracting gold from a goldmine whose every particle is crucial. In this article, we will explore What are the key steps in the data science process and how they contribute to the overall success of data-driven initiatives.
- Table of Contents
- Defining the Problem
- Data Collection
- Data Cleaning and Preparation
- Exploratory Data Analysis (EDA)
- Feature Engineering
- Model Building
- Model Evaluation
- Model Deployment
- Conclusion
- FAQs
What are the Key Steps in the Data Science Process?
Data science includes a series of techniques and methodologies focuses on extracting insights from large volumes of data. The steps in data science process provide a systematic approach to solving complex problems and making informed decisions based on data analysis.But before we begin to unreveal what is data science process and what are the steps of the data science process let’s qucikly go through the Mistakes.
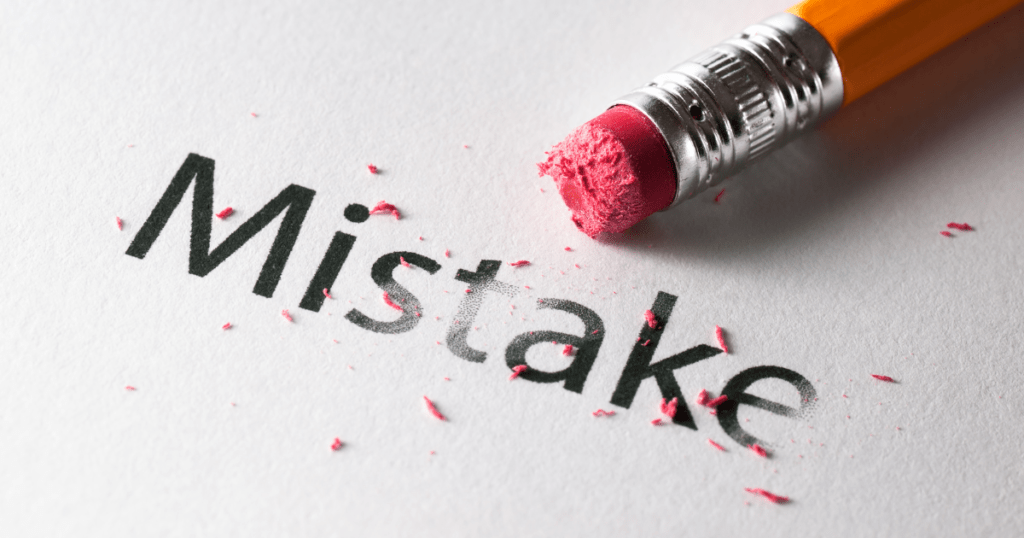
Mistakes While Building the steps in data science process!
Here are some common mistakes people make while building the steps in the data science process:
- Skipping proper problem definition and goal setting.
- Neglecting to collect comprehensive and relevant data.
- Failing to address data cleaning and preprocessing adequately.
- Overlooking the importance of exploratory data analysis (EDA).
- Neglecting feature engineering and selection.
- Rushing into model building without proper evaluation.
- Neglecting the deployment and maintenance aspects of the model.
These mistakes can hinder the effectiveness and success of the data science process, impacting the quality of insights and outcomes obtained.
1. Defining the Problem
Before diving into the What are the steps of the data science process, it is essential to clearly define the problem statement. This involves understanding the business objectives, formulating specific questions to be answered, and setting measurable goals for the project.
2. Data Collection
Once the problem is Figured out, the next process is to cater relevant data. Data can come from various sources, such as databases, APIs, web scraping, or even manual entry. It is important to ensure that the collected data is accurate, comprehensive, and representative of the problem domain.
3. Data Cleaning and Preparation
Raw data often contains errors, missing values, or inconsistencies that can adversely affect the analysis. In this steps in data science process, data scientists clean the data by removing duplicates, handling missing values, and transforming the data into a suitable format for analysis.
This process ensures that the data is reliable and ready for further exploration.
4. Exploratory Data Analysis (EDA)
EDA involves examining the data to uncover patterns, relationships, and hidden insights. Data visualization techniques, such as charts, graphs, and statistical summaries, are used to gain a better understanding of the data’s characteristics. EDA helps identify outliers, trends, and potential variables that may impact the analysis.
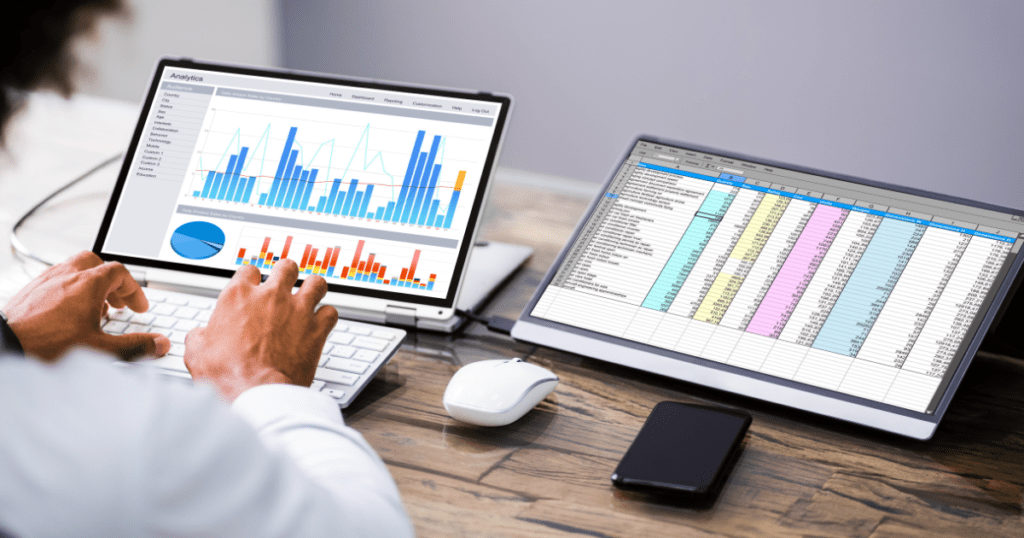
5. Feature Engineering
One of the steps in data science process is Feature engineering. This is the process of creating new features or transforming existing ones to enhance the predictive power of machine learning models.
It involves selecting relevant variables, scaling features, encoding categorical variables, and creating derived features that capture meaningful information from the data.
6. Model Building
Once the data is prepared and features are engineered, the one of the next steps in data science process is to build predictive models. Various algorithms and techniques, such as regression, decision trees, or neural networks, are employed to train models on the data. The choice of the model depends on the nature of the problem and the type of data available.
7. Model Evaluation
If you’re really looking that what are the key steps in the data science process is that After training the models, they need to be evaluated to assess their performance and generalization capabilities. Metrics such as accuracy, precision, recall, and F1 score are used to measure the model’s effectiveness. Cross-validation techniques help ensure that the model performs well on unseen data.
8. Model Deployment
Once a satisfactory model is developed, it needs to be deployed in a production environment. This involves integrating the model into existing systems, creating APIs for real-time predictions, or developing user interfaces for end-users. Steps in data science process that is Model deployment requires careful testing, monitoring, and ongoing maintenance to ensure optimal performance.
Conclusion
We have covered almost all the possible steps in data science process and questions like what are the steps of the data science process but if you still have the question in your mind that What is the Data science process then The data science process is a structured approach to extracting insights from data.
From defining the problem to deploying the model, each step plays a vital role in the overall success of a data science project.
By following this process, organizations can unlock the full potential of their data and drive data-driven decision-making.
FAQs
Q1: How long does the data science process typically take?
The duration of the data science process varies depending on the complexity of the problem, the availability and quality of data, and the resources allocated to the project. It can range from a few weeks to several months.
Q2: Is coding knowledge necessary for data science?
Yes, coding is an integral part of data science. Proficiency in programming languages such as Python or R is crucial for data manipulation, analysis, and building machine learning models.
Q3: Can the data science process be applied to any industry?
Yes, the data science process is applicable to a wide range of industries. From finance and healthcare to marketing and e-commerce, data science has the potential to drive insights and improvements in various sectors.
Q4: What is the role of domain knowledge in the data science process?
Domain knowledge is valuable in understanding the problem context, identifying relevant variables, and interpreting the results. It helps data scientists ask the right questions and make informed decisions during the analysis.
Q5: How can businesses benefit from the data science process?
By leveraging the data science process, businesses can uncover valuable insights, make data-driven decisions, optimize processes, improve customer experiences, and gain a competitive edge in the market.
In conclusion, the data science process comprises several key steps, from problem definition to model deployment. Each step contributes to the overall success of a data-driven project. By following a systematic approach, organizations can unlock the power of data and make informed decisions to drive growth and innovation.