What Are The Different Types Of Statistical Methods: Let’s begin with a very simple explanation of what statistical methods are! Statistics is the backbone of modern research and decision-making processes across various disciplines. From healthcare to economics, from psychology to engineering, statistical methods help us make sense of data and draw meaningful conclusions.
However, with a myriad of statistical tests available, it can get quite confusing as to who to who and what is which, right? ENTER, ZELL! Let us hand-guide through this simple and direct blog on What Are The Different Types Of Statistical Methods? How Do I Choose The Right Statistical Test? – So the next time you are caught in a data vex, you know which statistical method to summon.
What Are The Different Types Of Statistical Methods?
Before that – let us tell you what statistical analysis is. Statistical analysis, often referred to simply as statistics, involves the collection, organization, analysis, and interpretation of data based on established principles. This process helps to identify patterns and trends within the data.
The field of statistical analysis has broad applications across various domains, including academia, business, social sciences, genetics, population studies, and engineering, among others. Now onto our next step, what are the different types of statistical methods?
Main types of statistical analysis
There are three major types of statistical analysis: descriptive, inferential, and associational.
Descriptive Statistical Analysis: Descriptive statistics is the simplest form of statistical analysis, using numbers to describe the qualities of a dataset. It helps condense large datasets into simpler, more interpretable forms. This type of analysis employs data visualization tools such as tables, graphs, and charts to facilitate understanding and interpretation. However, descriptive statistics do not allow for making conclusions beyond the data presented; they merely represent the data, providing a basis for further analysis.
Inferential Statistical Analysis: Inferential statistical analysis involves making inferences or drawing conclusions about a larger population based on findings from a sample group. This method helps researchers identify differences among groups within a sample and validate generalizations about a population.
Associational Statistical Analysis: Associational statistics are used to make predictions and identify causation by examining relationships among multiple variables. This advanced form of analysis determines whether inferences and predictions about one dataset can be made based on the characteristics of another.
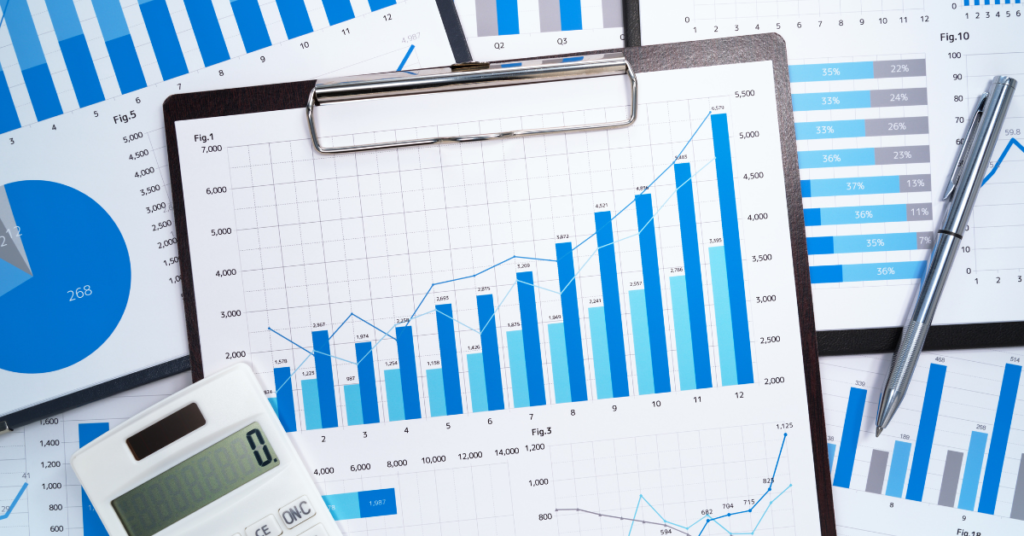
Other types of statistical analysis
In addition to the main types, there are several other important types of statistical analysis:
- Predictive Analysis: Predictive analysis uses statistical algorithms and machine learning tools to forecast future events and behaviors based on historical and current data trends. This method employs techniques such as data mining, big data, predictive modeling, artificial intelligence, and simulations.
- Prescriptive Analysis: Prescriptive analysis guides decision-making by suggesting the best course of action from several alternatives. It uses tools such as graph analysis, algorithms, machine learning, and simulations.
- Exploratory Data Analysis: Exploratory data analysis (EDA) helps data scientists identify patterns and trends within a dataset.
- Causal Analysis: Causal analysis seeks to understand why events occur, identifying the underlying factors that lead to specific outcomes.
What Are The Statistical Tests For Different Types Of Data?
Choosing the correct statistical test is essential for accurately analyzing your data. Different types of data require different statistical tests, each designed to handle specific data characteristics; here is a glimpse. Also, it is very very important to know the types of data is the first step in selecting the appropriate statistical test:
- Continuous data: Numerical data that can take any value within a range (e.g., height, weight, temperature).
- Categorical data: Data that can be divided into distinct groups or categories (e.g., gender, race, yes/no responses).
- Ordinal data: Categorical data with a meaningful order but no consistent difference between categories (e.g., ranks, survey ratings).
- Count data: Data representing counts of occurrences (e.g., number of visits to a website).
What are the statistical tests for different types of data? Let’s find out here.
Parametric Tests
These tests assume that the data follows a normal distribution:
- Independent t-test: Compares means between two independent groups.
- Paired t-test: Compares means within the same group at different times.
- ANOVA (Analysis of Variance): Compares means among three or more groups.
- Pearson Correlation: Measures the strength and direction of the relationship between two continuous variables.
- Linear Regression: Models the relationship between a dependent variable and one or more independent variables.
Nonparametric Tests
These tests do not assume a normal distribution and are used when data doesn’t meet parametric test assumptions:
- Mann-Whitney U Test: Compares differences between two independent groups.
- Wilcoxon Signed-Rank Test: Compares differences within the same group at different times.
- Kruskal-Wallis Test: Compares differences among three or more groups.
- Spearman Correlation: Measures the strength and direction of the relationship between two continuous variables.
Statistical Tests for Categorical Data
- Chi-Square Test for Independence: Assesses whether two categorical variables are related.
- Fisher’s Exact Test: Used instead of the chi-square test when sample sizes are small.
Statistical Tests for Ordinal Data
- Mann-Whitney U Test: Compares differences between two independent groups.
- Wilcoxon Signed-Rank Test: Compares differences within the same group at different times.
- Kruskal-Wallis Test: Compares differences among three or more groups.
- Spearman Correlation: Measures the strength and direction of the relationship between two ordinal variables.
Statistical Tests for Count Data
- Poisson Regression: Used when modeling count data that follows a Poisson distribution.
- Negative Binomial Regression: Used when count data is over-dispersed, that is, the variance is greater than the mean).
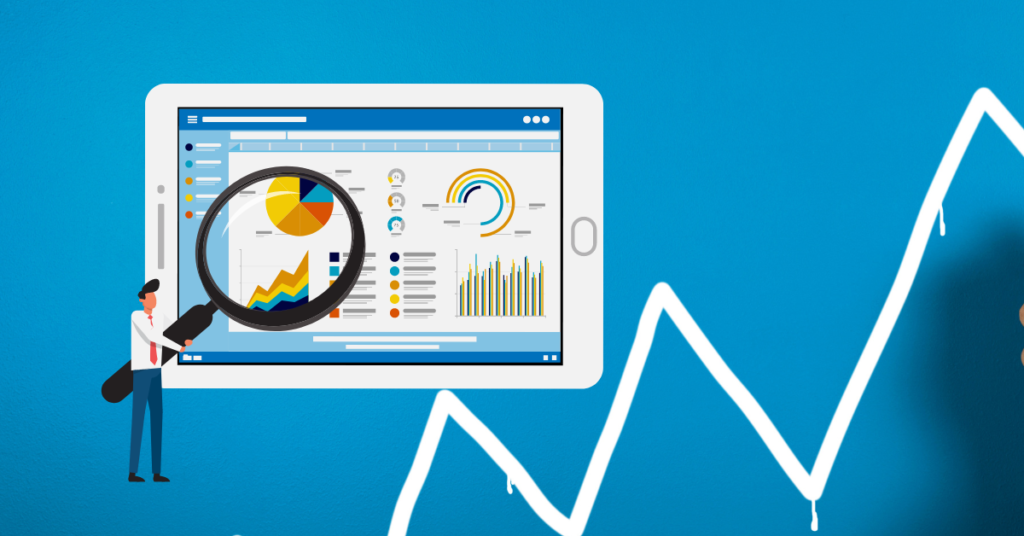
How Do I Choose The Right Statistical Test?
Choosing the right statistical test is crucial for getting accurate and reliable results. Here are some key factors to consider when making your selection so the next time you don’t have to wonder on: how do I choose the right statistical test!
- Identify the type of data: Determine whether your data is continuous, categorical, ordinal, or count.
- Determine the number of groups: Identify how many groups or variables you are comparing.
- Check assumptions: Assess whether your data meets the assumptions required for parametric tests (e.g., normality, homogeneity of variance).
- Select the test: Based on the type of data, the number of groups, and the assumptions, choose the appropriate test from the lists above.
What Is The Difference Between Parametric And Nonparametric Tests?
In the previous section, we mentioned parametric and nonparametric tests, but what is the difference between parametric and nonparametric tests? They differ in their assumptions about the distribution of the data and the parameters being estimated.
Parametric Tests
- Assume that the data follows a specific probability distribution, often the normal distribution.
- Estimate population parameters such as means and variances.
- Examples include t-tests, ANOVA, Pearson correlation.
Nonparametric Tests
- Do not make assumptions about the underlying distribution of the data.
- Focus on the ranks or orderings of data points rather than their actual values.
- Suitable for non-normally distributed data or when sample sizes are small.
- Examples include Mann-Whitney U test, Wilcoxon signed-rank test, Spearman correlation.
To learn more about Statistical methods, data science and more, reach out to us at Ze Learning Labb.